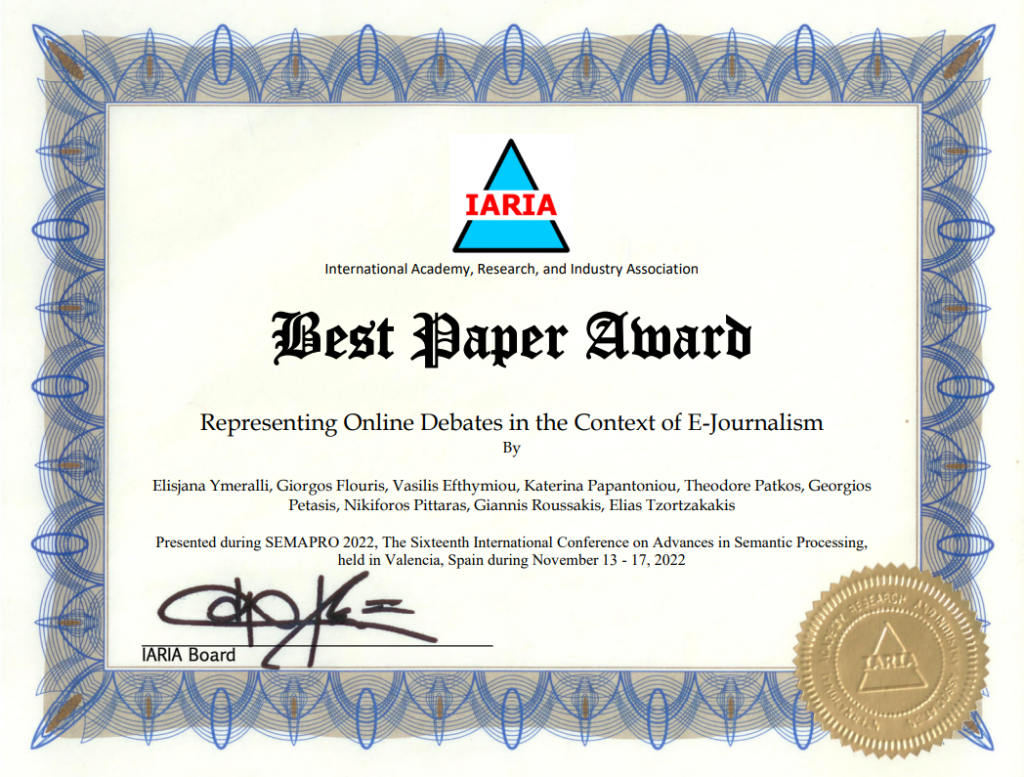
The work entitled “Representing Online Debates in the Context of E-Journalism” that has taken place in the context of DebateLab project has been awarded with the Best Paper Award at the SEMAPRO 2022, The Sixteenth International Conference on Advances in Semantic Processing conference held in Spain in November.
For more information on paper see here.
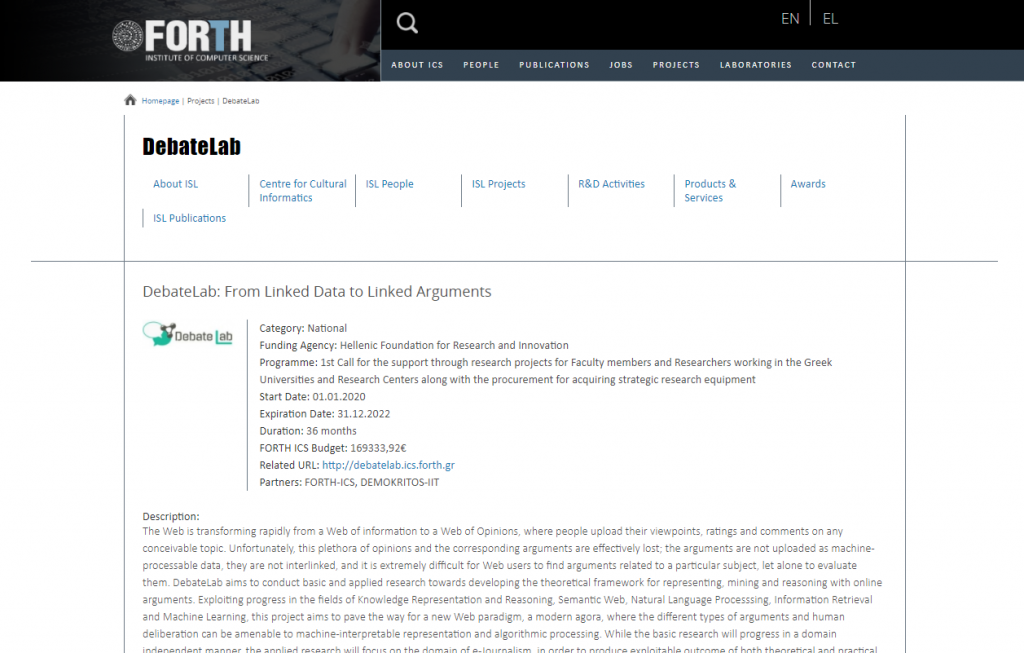
The DebateLab project is conducting research towards representing, mining and reasoning with online arguments. The goal of DebateLab is to offer a suite of tools and services that will assist both the work of the professional journalist in accomplishing everyday tasks (e.g., writing, archiving, retrieving articles), as well as the activity of the ordinary Web user (reader) who wishes to be well-informed about topics or entities of interest (e.g., persons, locations, events).
DebateLab is conducted at FORTH-ICS in collaboration with SKEL-NCSR “Demokritos”, by a balanced consortium comprising senior, principal and postdoctoral researchers, PhD students and a software engineer.
For more information, visit the FORTH website here.
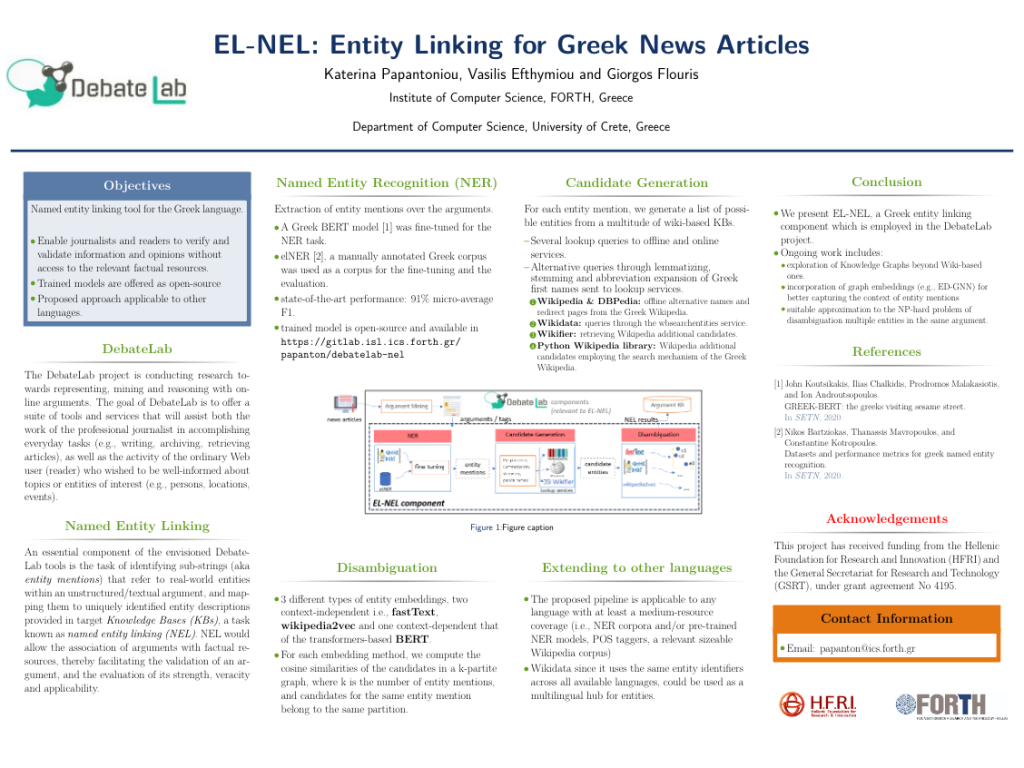
This poster presents the work that has be done in the context of the DebateLab project, by briefly describing the pipeline of the EL-NEL implementation for non-English language, the modular architecture and alternative options for each component of EL-NEL. The aim of this work is to present the process followed for identifying sub-strings (entity-mentions) within an unstructured textual argument and then mapping them to uiniquely identified entity descriptions provided in the Knowledge Bases (KBs).
For more information, see the paper here.
A demo is also available, here.
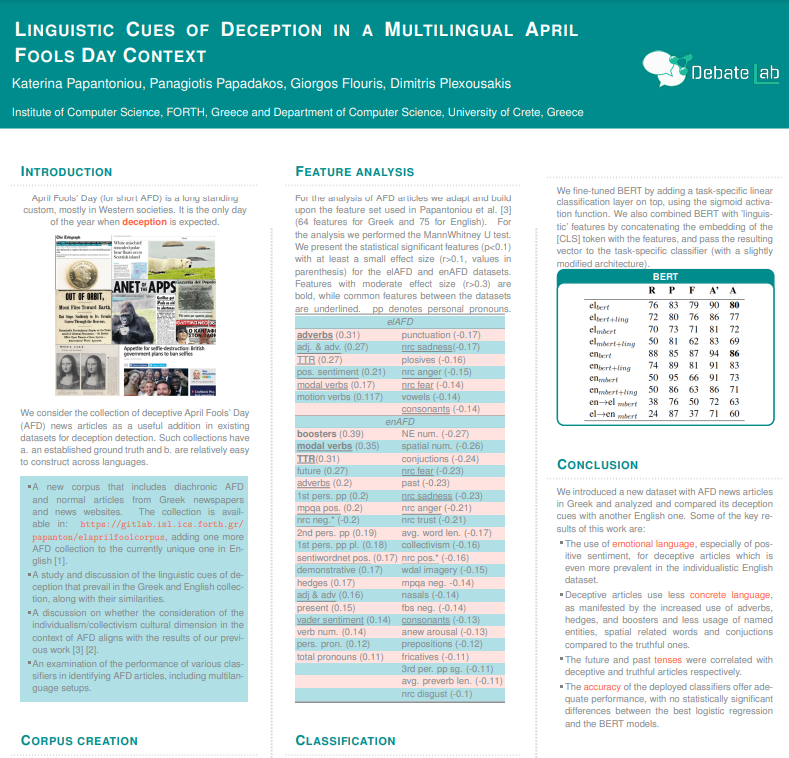
This work considers the collection of deceptive April Fools’ Day (AFD)
news articles as a useful addition in existing datasets for deception detection tasks. Such collections have an established ground truth and are relatively easy to construct across languages. As a result, we introduce a corpus that includes diachronic AFD and normal articles from Greek newspapers and news websites. On top of that, we build a rich linguistic feature set, and analyze and compare its deception cues with the only AFD collection currently available, which is in English. Following a current research thread, we also discuss the individualism/collctivism dimension in deception with respect to these two datasets. We also build classifiers by testing various monolingual and crosslingual settings. The results showcase that AFD datasets can be helpful in deception detection studies, and are in alignment with the observations of other deception detection works.
For more information, see the paper here.
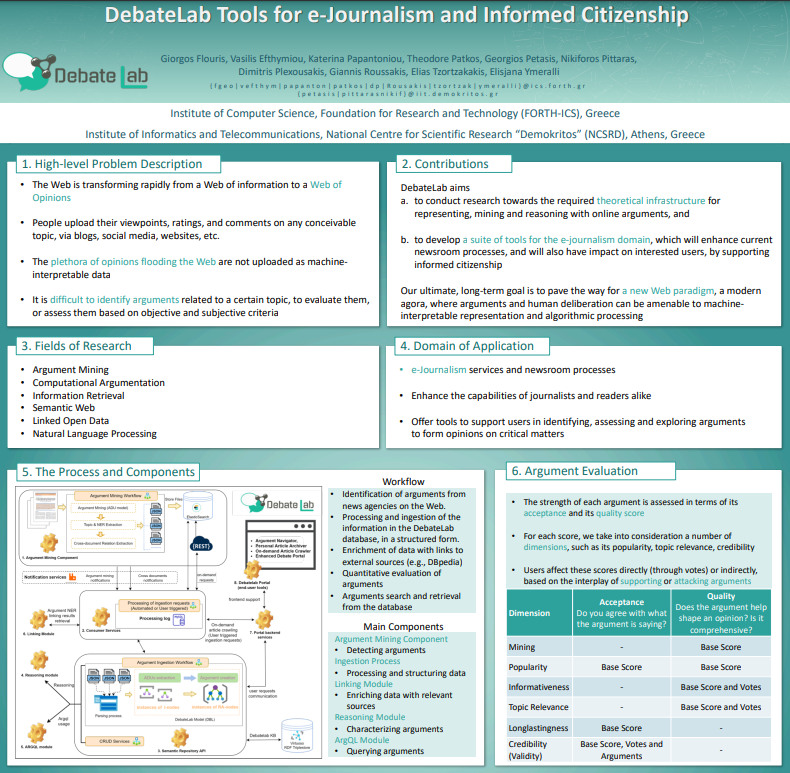
This work describes DebateLab, a project which aims to conduct research towards developing the theoretical infrastructure for mining, representing and reasoning with arguments found online, while delivering a suite of tools and services supporting the uptake of the related technologies. DebateLab will pave the way for a new Web paradigm, where the different types of arguments and human deliberation will be amenable to algorithmic processing and machine interpretable representation. Towards this, DebateLab analyzes articles with argumentative content to provide tools that will be useful for the professional journalist, but also for users who want to be better informed regarding public debates.
For more information, see the paper here.
Belief Revision and Argumentation Approaches to Support Commonsense Reasoning.
Belief Revision and Argumentation Approaches to Support Commonsense Reasoning Allowing artificial agents to model and reason about commonsense phenomena is one of the major problems of AI research since its conception. In this talk, I will present recent (and partly unpublished) research that has been performed in the Symbolic AI group of FORTH-ICS, which connects the fields of commonsense reasoning with the fields of belief revision and computational argumentation. The talk will be split in two parts.
In the first part, Giorgos Flouris consider how belief revision can support agents in their reasoning about events, their effects, and their preconditions, which is one of the main desiderata of commonsense reasoning. Event Calculus is a powerful non-monotonic language for allowing this kind of reasoning, enabling the modelling of commonsense phenomena in causal domains, but no belief revision methods for Knowledge Bases modelled using Event Calculus exist. As a result, agents cannot handle unexpected observations, i.e., observations that are inconsistent with the agent’s perceived world view, as dictated by the events that the agent has witnessed (and their expected effects). To address this problem, I will describe work that adapts well-known ideas from belief revision to apply on Event Calculus theories, proposing a belief revision algorithm for Event Calculus that satisfies the main principles of belief change.
In the second part of this talk, I will present recently-proposed extensions of the standard frameworks for Computational Argumentation, which are more suitable for reasoning about the scope of arguments, their exceptions, and their relevance for specific contexts, an important concept of commonsense and non-monotonic reasoning. In the proposed extensions, arguments are equipped with a domain of application, referring to the objects in the universe that each argument applies to. Appropriate semantics for these frameworks are presented, through which attacks among arguments limit their domain of application, rather than invalidating them altogether (as in classical Computational Argumentation settings). Thus, the proposed models inherently support the notions of exception and scope of arguments.
The presented work has been published in Commonsense-17, AMAI and IJCAI-21.
This research was supported by the Hellenic Foundation for Research and Innovation (H.F.R.I.) under the “1st Call for H.F.R.I. Research Projects to support Faculty Members and Researchers and the procurement of high-cost research equipment” grant (Project Number: 4195).